
Mostly, these techniques are based on statistical characteristics of the outcome set. 2005 Jager 2008) have been proposed to increase the precision and recall of the results. Many pre- and post-processing techniques (Jaroszewicz and Simovici 2002 Goethals et al. However, it is not uncommon for data mining to lead to an unmanageable number of outcomes with a great deal of co-related results that only challenge human understanding. 1996 Berry and Linoff 1997 Kantardzic 2003). Nonetheless, data mining experts are required to navigate and prune the results, looking for useful, unexpected and promising patterns and associations raised in the process (Fayyad et al. There is concrete evidence of the usefulness of data mining of large datasets. Results for various data mining techniques have been promising, when observed at a small scale. In this scenario, data mining has come to be regarded as a useful technique with which to synthesize a large amount of data into more condensed sets of knowledge. To gain insights from such large amounts of data, researchers and businesses have developed techniques to manipulate this humanly unmanageable amount of information. Data can come from different sources, in different formats and even with different structuring.
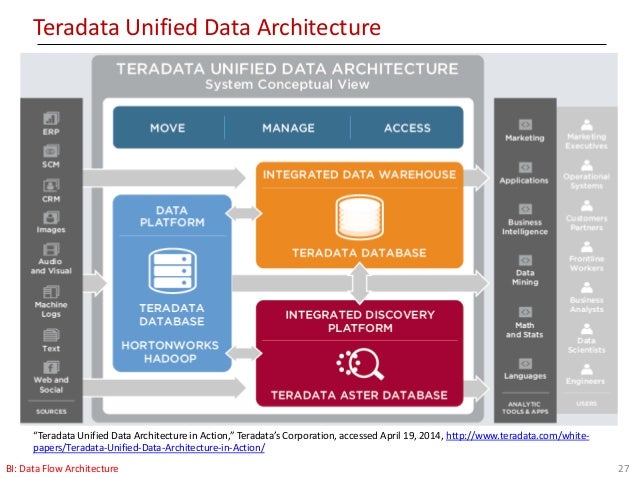
New information is created every day and brought to public notice through the Internet or company databases.
